Maritime businesses rely on the trusted information at the right time. It’s crucial for pre-fixture analysis, charter party claims, route optimisation, CII reporting, benchmarking against industry performance and many other activities. Thousands of dollars may be lost on every voyage if the optimisation relies on an inferior performance model.
So, when choosing a vessel performance model, a proper assessment of its quality is key. The challenge is that assessing the quality of vessel performance models is fraught with pitfalls. In many cases, model quality assessment methods are insufficient or even misleading, which creates a real risk that critical business decisions are made on shaky foundations.
To avoid this, we have used our expertise highlight five things to avoid when selecting voyage performance software, listed below. The key learning is this; accurate and relevant measures of model quality are every bit as important as the models and must come before we can confidently apply vessel performance models to any use case.
Mistake #1: Not measuring the ability to generalise
Performance models are trained on historic or past data. Ideally, the models are retrained frequently, such as after each newly completed voyage. But there will always be some degree of separation between the training data, and the data served to the model at ‘crunch time‘ and used to predict performance in real-life applications.
For this reason, it is critical to assess what is referred to as the model’s generalisation ability. That is, how well can the model predict performance in new conditions? To that end, the model should be tested on data it has not trained on – that is, conditions and speeds that it has not interpreted before.
Mistake #2: Treating tests as single results and not collectively
The inputs and outputs that make up the data that vessel performance models are trained on are not deterministic, but stochastic; which means they are random variables. Each measurement is an observation of a random process. Every time it is observed, a different number will be obtained.
As with all tests based on stochastic data, the test results will also be random. If only a single test is done, then an incorrect conclusion can be drawn. If, however, the average result of five tests is used, the conclusion is much less susceptible to the randomness in the data and the conclusion drawn on it is therefore much less likely to be wrong.
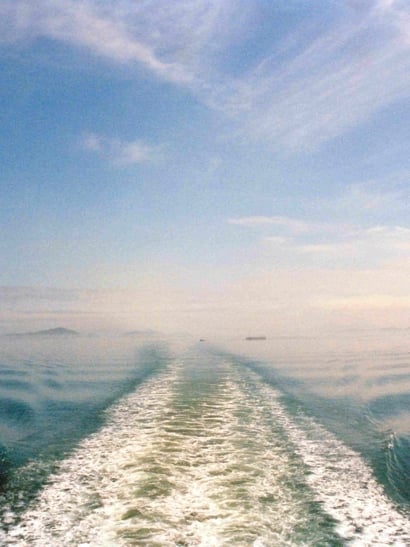
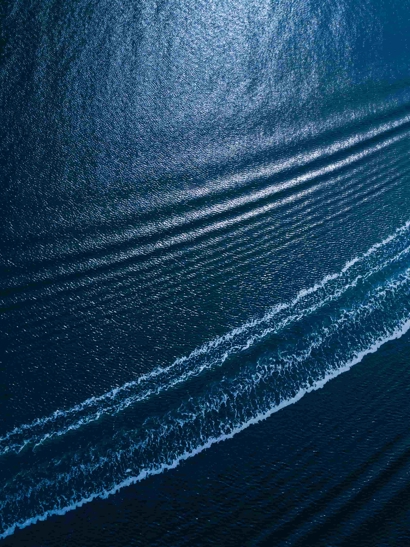
Mistake #3: Ignoring input noise
To predict how much power is needed to achieve a certain speed through water, many conditions must be factored in; currents, wave spectrum, wind speed and direction, draft and trim, sea depth, rudder angle and sea surface temperature, for example.
Speed through water is notoriously hard to pin down. Oceanographic current estimates are uncertain and speed logs can be unreliable, requiring careful and frequent calibration and maintenance.
If the inputs are assumed to be noise-free (deterministic), the quality assessment of the model will, to a degree, be confused by the effects of the variation in the inputs, since they are stochastic (noisy).
Mistake #4: Ignoring output noise
The output data is often affected by various sources of randomness and bias that can be hard to deal with. The output in machine learning is often called ‘the target’, which makes it obvious that if the output data used to train the model is bad, the resulting model will be bad – no matter how perfect it could be if only the data behaved.
A highly risky approach is to ignore these potential issues and simply train on all the available output data. Although this is sometimes done, it should be avoided.
"Thousands of dollars may be lost on every voyage if the optimisation relied on an inferior performance model."
Mistake #5: Using the wrong quality metric
The observed differences between model predictions and reported power or fuel consumption are stochastic, random variables. The higher the quality of a performance model, the smaller these differences will be.
The differences will, of course, never be zero – no model is perfect - and the data, both inputs and outputs, are noisy, so how should differences of varying magnitude be interpreted, for the purpose of assessing model quality? This comes down to a choice of metric for summarising all the observed differences into one model quality KPI. A good metric reflects the true distribution of the differences.
Want to know more?
If you would like a copy of the full knowledge paper please send your request to us by emailing marketing@i4-marketing.com.
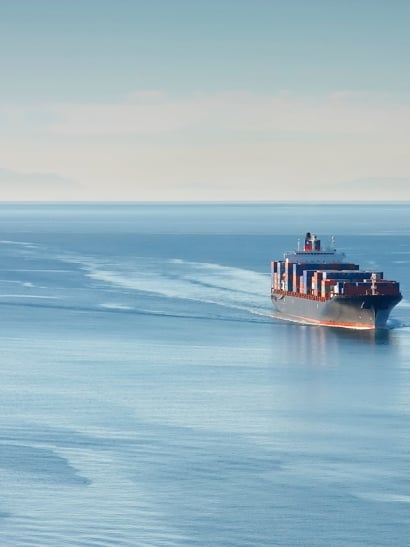